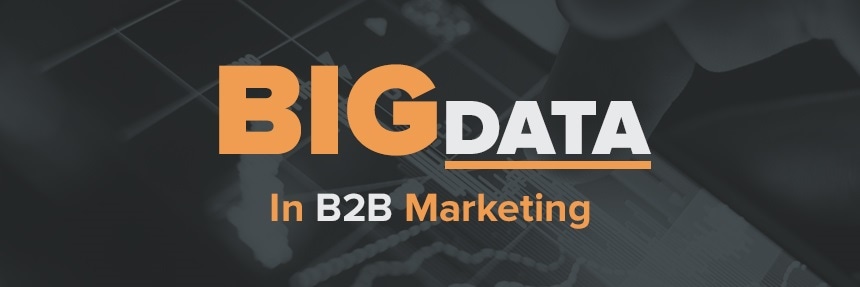
Updated for January 2020
What Is Big Data?
Today’s businesses are dealing with more data than ever. Data from financial transactions, CRM systems, qualitative data, social media interactions, analytics platforms... the list goes on.
In the midst of all of this data, companies need novel ways to capture data, verify its accuracy, and use it to develop models that can inform strategic decisions. Usually, the most valuable data of all concerns a single, ever-fluctuating commodity: Your customers.
What Kind of Data Do Companies Collect?
The B2B sales cycle is getting longer and more complex — In a recent survey 76% of scientific purchasers said that online publications influence their decision to buy. More decision-makers are participating in consensus sales involving multiple stakeholders, with the optimum size of a purchasing team being between 4-5 people... Any more and a deal becomes increasingly subject to decision paralysis!
71% of B2B technical purchases involve a team of 4 or more people - AZoNetwork purchaser's survey
The end result for specialist enterprises, especially in the scientific industry, is many months spent cultivating a single sale.
Collecting, interpreting and operationalizing customer data is one way to be proactive in the face of lengthening sales cycles. Knowing more about potential customers, the business landscape they face, and the market helps:
- More informed product development with greater likelihood of market penetration.
- Deeper understanding of customers’ needs and the best marketing strategies to use.
- Ultimately, a sales cycle with greater opportunities to accelerate and inspire action.
When sales and marketing departments have to prioritise time and resources, they need a system which automatically scores and qualifies their leads.
In architecting a complete picture of its customers, a business draws on two broad data types:
Explicit Characteristic Data
Characteristic data begins with informed assumptions about the ideal customer: Who they are, where they work, and what their background is. This framework is gradually filled out using verifiable, quality data from real customer records. In addition to things like education, age and job title, characteristic data might include details on which publications and websites influence a customer.
Intrinsic Behavioral Data
Behavioral data shows customers in action. It might be real-time information from your website, showing how users interact with it and suggesting how you can make it more compelling. Or, it may come from third-party platforms such as those that track user interactions with your advertisements across the internet.
Characteristic data and behavioral data combine to provide authentic insights about real prospects and customers. A real world example would be in lead scoring - Imagine you have two leads:
Charlie is a CEO of his own start-up company and Vicky is a VP of Research in a government laboratory. To start with, they both look like reasonable leads. Let's have a look at how lead scoring works and how we could automatically evaluate and prioritise which lead to work on first.

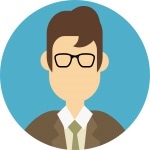
Vicky the VP and Charlie the CEO
Vicky the VP ticks all of the boxes in terms of characteristic data: Size of company, job title, target country, budgets and working within your product's application/research areas. A great characteristic fit! However, her behavioural data shows that she has only downloaded a single PDF (filled in a form) of an application note.
In this instance, the explicit characteristic data would be 60/60 (a 100% characteristic fit) but the intrinsic behavioral data would only be 7/60 (a 12% behavioural fit) - There was a general interest, but no real purchase intent. Overall, the score was 67/120, what appeared to be a good lead on the surface was only a 56% fit at this moment in time. She may come back and request a quote for big order in the future!
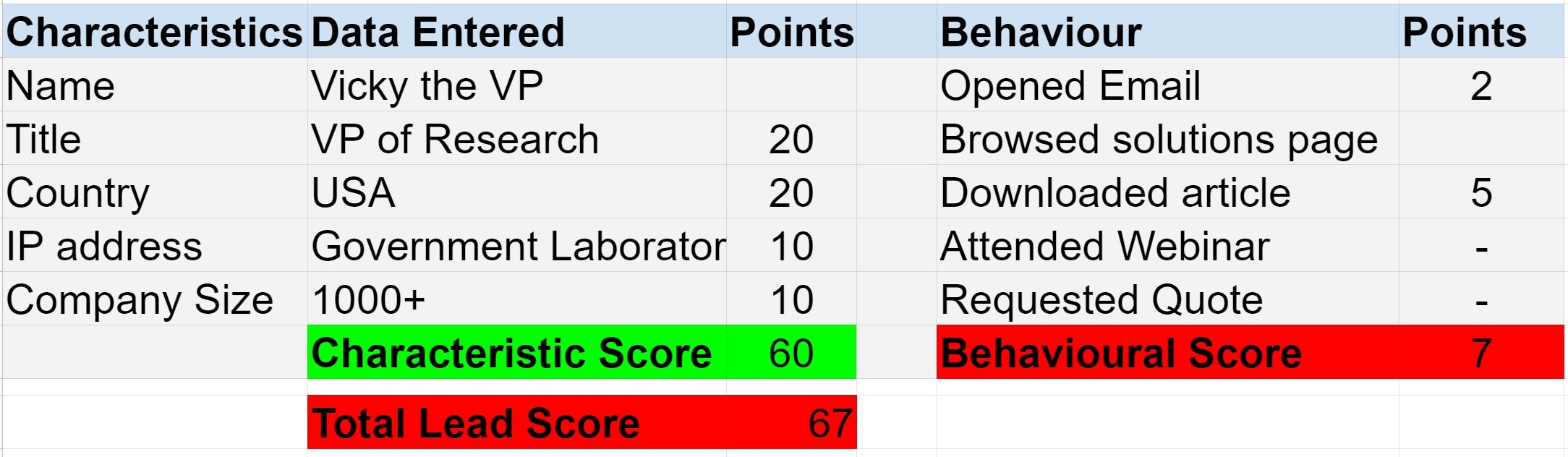
Conversely, Charlie the CEO has characteristic data that only scores 30/60 (a 50% characteristic fit) however he has become really engaged with the webinar, solution content and has decided to request a quote. This gives him a behavioural score of 72/60 and an overall rating of 102/120 or 85%.
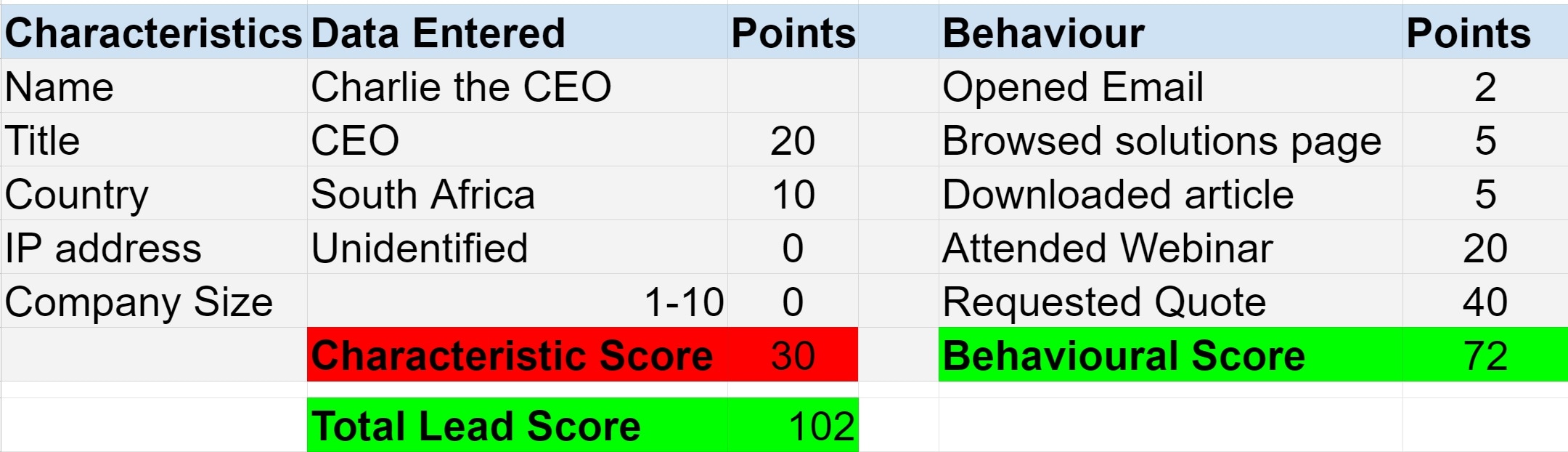
Two very good leads and whilst Charlie the CEO should be passed to sales immediately as an SQL, Vicky the VP should be nurtured as a Marketing Qualified Lead and should receive related information to her application of interest. This simple example demonstrates how lead scoring can be scaled. Any combined score over say 80% should go through as a Sales Qualified Lead, you can always modify this threshold later.
Lead scoring models will vary from business to business depending on what you value in a prospect. They should be reviewed every few months and you should actively seek feedback from your sales team on the quality of leads being passed through and tweak your model accordingly.
How Do Companies Use Data for Marketing?
Businesses use data for two major purposes:
- To track the progress of individual prospects and customers through the buyer journey.
- To provide timely, relevant, and personalized customer touch-points during that journey.
In some cases, applying data in a deft and unobtrusive way can significantly improve the value-per-visit of an existing customer. For example, user data could be synthesized to develop better product recommendations. These recommendations are then used to enhance the user experience which, in turn reduces bounce rate and increases dwell time. Both of these are applications of predictive analytics.
Even in this simple example, many factors go into calculating the best recommendation:
Above shows how we enhance user experience by providing very specific related alloy content to the reader's interests.
- Past browsing behavior of the individual user.
- Time of year and its bearing on buying patterns.
- Current sales and special offers that might apply.
- Other factors, including long-term sales strategy.
One of the most powerful and controversial applications of data science in ecommerce involves user tracking. Advanced tracking is used in retargeting, the display of ads on other sites to users who have interacted with your site before.
In a comprehensive retargeting strategy, ads are purchased on other platforms that might induce a user to re-vist an application, return to a product page visited previously, or sign up for a webinar the user only briefly read about.
So next time you are on Youtube and an Ad pops up from a niche site you've visited recently, that's a savvy marketer trying to reel you back in.
Ask about Google Ads Remarketing
How Do Users Feel About Tracking?
There has been a shift in sentiment in recent years as to how users feel about an application's ability to track their behaviour. One study, which specifically looks at user comfort levels when tracking their location data, shows that now only 15% of users are uncomfortable with sharing their location data.

It would seem this is mainly a trust issue. As long as you are transparent about how you use their data (i.e. to improve their user experience, provide them with more relevant content etc.) most users will be happy to proceed.
How Do Privacy Laws Impact Big Data?
In the United States, privacy regulations are generally on the side of business. There are only a few major exceptions; for example, businesses must strictly control data pertaining to younger users to comply with the Children’s Online Privacy Protection Act. Those sending commercial emails must adhere to the disclosure requirements of the CAN-SPAM Act.
In the U.S., businesses are generally free to use data collection and tracking methods like cookies at their discretion. For firms operating internationally, there are several special cases requiring certain methods to be limited or disclosed to the end user.
For example:
What’s the Best Way for Businesses to Collect Data?
According to a global survey conducted by Microsoft, users are interested in both personalization and privacy. It showed 54% of consumers “expect brands to really know and understand them as people, and for communications to be tailored to their values and preferences.”
99% of Users are Willing to Share
What’s more, 99% of users are willing to share some personal information if they are asked and rewarded. Cash was found to be the most persuasive reward, while loyalty points were among the least — but a logical, persuasive, clear rationale for the exchange remained absolutely essential.
For both legal and PR reasons, brands should look for ways to be transparent and forthcoming about how information is collected and used. According to Pew, half of online Americans don’t know exactly what a privacy policy is for — so direct, simple language is key.
An Overview of Advanced Big Data Applications for Business
As the quantity of data grows, new techniques can be deployed; and with a robust data science function in place, it becomes possible to inform marketing with data in highly sophisticated ways. Let’s review some of the most effective.
Predictive analytics is the use of past customer data to predict future events. Data mining, machine learning, statistical analysis, and modeling can all be employed. It offers both consumer-focused and operations-focused applications; for example, pricing optimization, fraud prevention, and demand forecasting are all facilitated by predictive analytics and can save businesses millions.
IBM research shows ROI for predictive analytics projects as high as 250%, with the median ROI for such initiatives growing substantially over time.
Target Personas - A New Approach to a Classic Idea
Many aspects of marketing begin with fundamental assumptions about the customer. These include basic characteristics like professional background, education, age, gender and pain points. Over time, information from sales and marketing teams give these personas greater depth.
Analytics can streamline the process of refining personas. For example, tracking information can provide deeper insight into what publications influence particular users. Detailed data might lead to the development of granular “sub-personas” where only one had seemed to exist.
Hyper-Personalized Content - The Tactic That Helped Starbucks Add 10 Million Loyalty Members
Hyper-personalization describes any effort to adjust or structure customer-facing communication using data that applies personally to the user. One of the simplest, yet most effective forms of personalization is including the user’s name in an email subject line: This can raise open rates nearly 30%. With sophisticated data, hyper-personalization can closely simulate 1-on-1 communication.
The ubiquitous coffee shop Starbucks has had great success with hyper-personalization, but it can also apply to B2B enterprises. For example, B2B firms with multiple websites can consolidate user profile information to clarify customer behavior. The marketing team can design granular content focused on specific pain points to create a greater sense of 1-on-1 connection.
Behavioral Data for Lead Scoring
Lead scoring is a method of prioritizing follow-up by assigning quantitative values to each lead. The most valuable leads, for example, may be those who combine factors like a certain job title, buying authority, budget size, and exposure to a certain sequence of web content.
When leads reach a certain score, sales might contact them proactively. If they “stall” at a given score, other actions might be taken to move them along the buyer’s journey. The more data an enterprise has, the easier it will be to connect customers' actions to an effective response.
Scientific enterprises have the advantage of great fluency in the use and importance of data. To remain competitive, they must apply this insight to new analytical marketing technologies and techniques.